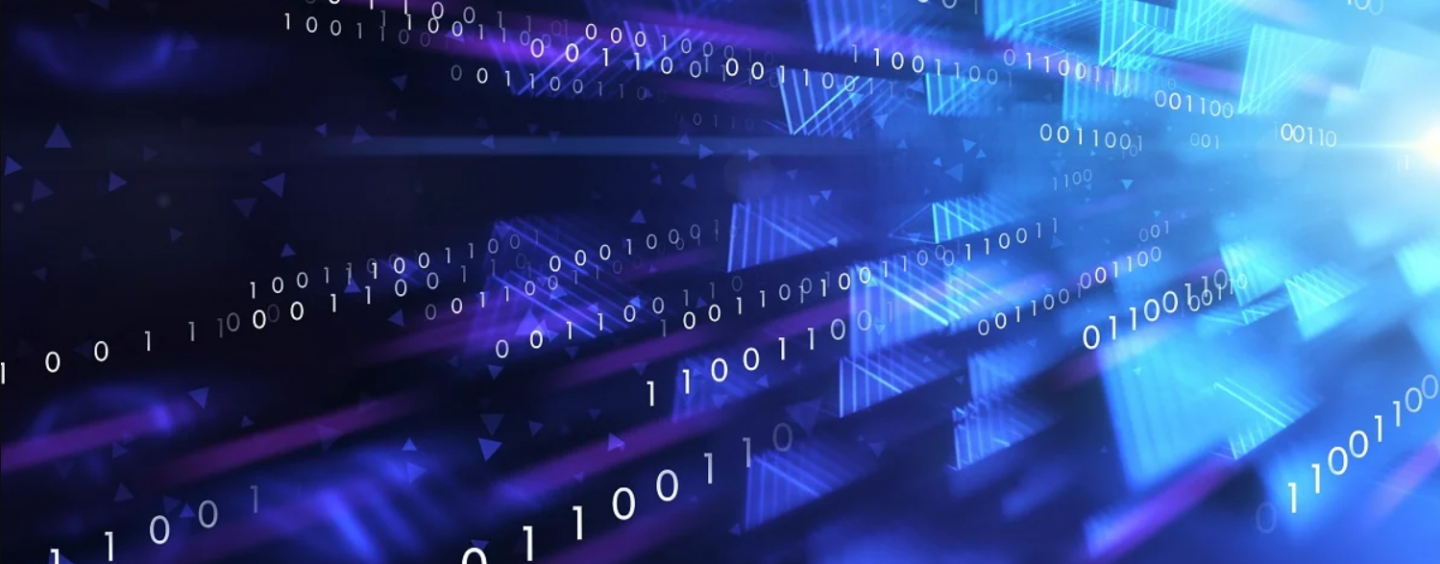
As business leaders look to capitalize on AI opportunities, they are asking, how to predict the future returns for AI projects. Where should the investment be targeted, and what kind of capabilities can enable better performance?
Assessing the future value of AI
When
we think what AI can do to the business, it mostly comes from multiple
well-marketed examples, coming from well-defined areas. Like, the best chess
player getting beaten by Artificial Intelligence. But problems like this, have
a definitive endpoint, like in chess. However, most problems in Fortune 500
companies do not have a definite outcome. Problem statements that these
companies deal with are, for example, will the next product launched, be
successful? But what’s the definition of success here? Similarly, another example
can be, how to improve customer experience in banking or similar sectors?
Most of these problems are vague and complex, and the
outcomes defined in multiple ways. So, the challenge comes in quantifying the
outcome on investment in something where the outcome itself is vague. To answer
this question, the most important thing to ask is whether we truly understand
the business problem that we want to solve. In other words, is the business
question well framed?
These are open-ended business problems and often use-cases where machine learning wasn’t in use before. For example, when we try to define payment fraud, there may be false positives and negatives that, attaining a specific ratio could define success. However, when we want to improve customer value for a wealth management client over 20 years, how do we get an answer for that. In this situation, it is not only vague but also an area where AI was not in use. To work in these areas, the very first thing to do is lock-in and frame precisely what the problem is.
Scaled
problem solving
When
Fortune 500 companies look at solving problems, they focus on solving them at a
scale that can have an impact not only at a functional area but on the overall
business and be sustainable. To get a deep understanding of what we are trying
to solve, framing and reframing the business problem is a critical
pre-requisite. Is it a problem of growth, is it a problem of inefficiency, or
is it a problem of a better experience for our consumers? What are we looking
to solve here?
When we talk about problem-solving at scale, there are three parts
to the solution, post problem framing. The first is, identifying the levers of
experience, growth, or inefficiency through advanced analytics/AI. The second
one is to apply an engineering mindset and identify elements required for the
AI initiatives to be successful at enterprise scale including setting up the
appropriate architectural elements, managing the data lineage, latency,
ingestion, obfuscation, and dev-ops.
Ultimately,
the algorithms are only as accurate and actionable as the underlying data. And,
if we have to do things sustainably, will the data be available, accessible and
accurate over a period of time. The third and final component is execution,
including design of experiments and human- centered learning. We also call this
the final mile of decision making.
Every decision that enterprises are talking about is
going to impact a human and is going to be made by a human. Do we understand
those human needs that need a solution? To solve problems at scale, we need
three elements to come together, i.e., better algorithmic sophistication,
better engineering, and a better understanding of human behavior.
The code to success
The big-picture approach rests on various factors. There
is no single way to get everything right. The ability to test and learn, the
ability to experiment, the ability to fail fast but pick up the learnings and
quantify the learnings are going to be very important.
Here is an example of a consumer goods company that made a remarkable transformation through AI. The approach the company took was to give every initiative a six-week time to show progress. What it allowed the company to do is become more rapid, not just in defining problems but also in defining the quantifiable measurement of success and progress. So, in 12 months, 30-40 different initiatives were executed to achieve Minimum Viable Outputs (‘MVO’) out of which 5 to 6 specific initiatives taken into organizational scale.
An ecosystem of innovation
What
we learned from the example quoted above was, for an initiative to be
successful, cross expertise teams need to work together. Projects are
successful when there is an inter-disciplinary approach, as opposed to a
multi-disciplinary framework. We further realized that the agile mode of
working was going to yield better results and finally documenting learnings and
quantifying the learnings increases the probability of success.
AI is a capability that helps us become more non-linear. A human
plus machine approach will almost always be substantially better than the most
intelligent human or the most sophisticated algorithm. The real value of
success is if we realize that AI is a supply chain of several cobs to come
together for customer experience to become better. When we look at the return
on investment, we need to be cognitivist to the point that many of these moving
parts need to come together for value addition. The process of defining and
measuring the ROI of AI is a journey with the opportunities to affect change on
business truly limitless.