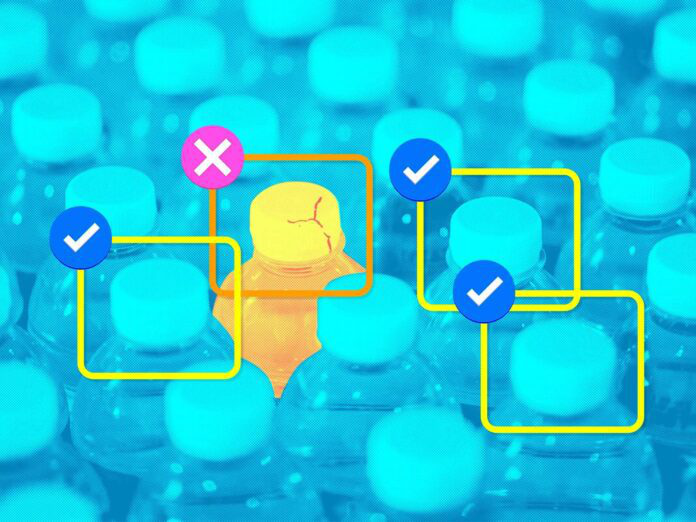
As industrial environments become increasingly digitized,
manufacturers are considering how Artificial Intelligence (AI) can be
implemented to automate processes that have previously required human
operators. To this end, quality control is one of the most important processes
within a manufacturing system, yet manual inspections often come with
substantial drawbacks as defects can easily be overlooked by the human eye.
Automating defect detection with AI-powered visual inspection can create a
reliable means of mitigating quality control errors even in complex
manufacturing environments.
Defect
Classification & Manual Visual Inspection Limitations
Operator conducted
Visual inspection is the method of looking for flaws or imperfections using the
naked eye and non-specialized inspection equipment. This approach is
commonly used by maintenance professionals in physical asset management across
industries and often serves as the final step in the manufacturing.
However, defects
can be easily missed by the naked eye, even for experienced operators. Even
small oversights can have wide reaching and costly consequences, resulting in
lower component quality or a defective final product that may be rejected. With
more complicated manufacturing systems, the number of defects will likely
increase.
Engineers are
continuously developing new ways to automate repetitive manual activities and
having an automatic system to detect abnormalities and discrepancies in
elements is critical for the quality inspection process. AI is are the
forefront of these efforts, as it enables the automation of sophisticated yet
respective activities.
The Concept of
AI-based Visual Inspection Explained
While AI offers
endless process automation potential, its initial benefits are often overstated
as AI systems require careful implementation to ensure they operate
successfully. In this case, AI is able to take on the task of automated visual
inspection processes through the power of machine learning (ML), or learning by
example In order to build an artificial neural network.
·
Artificial
Intelligence: Simulates human intelligence to automate a set behavioral
patterns.
·
Machine
Learning: Algorithms that build models based on sample data in order to make
predictions, decisions, or improvements without being explicitly programmed to
do so.
·
Deep
learning: Artificial neural networks in which multiple layers of processing are
used to extract progressively higher level features from data.
Through a process of extracting common patterns between labeled examples of specific data points. ML systems then translate case scenarios into a systemic equation, building a neural network with labeled examples and interconnected data points. AI systems rely their neural network to engage in deep learning, ultimately aiding in the classification of future data.
Benefits of AI
Enabled Industrial Defect Detection
The following are
some of the ROI that businesses can expect to see from implementing and AI
powered defect detection:
·
It
improves production yield while lowering waste.
·
Customer
satisfaction rises as product quality rises.
·
Warranty
claims and after-sales defects are drastically minimized.
·
Locating
parts and ensuring that product assembly is completed efficiently.
·
It is
now possible to detect faults that are unpredictable and varied.
·
Classifying
problems and parts improved process control.
How to Integrate/Implement AI Visual Inspection Systems
With enough data, the neural network will eventually detect
defects without any additional instructions. Deep learning-based visual
inspection systems are good at detecting defects that are complex in
nature. They not only address complex surfaces and cosmetic flaws—but also
generalize and conceptualize the parts’ surfaces.